Artificial Intelligence (AI) isn’t just transforming operations, it’s reshaping how people behave and make decisions. For C-level executives, particularly those in digital leadership roles, understanding how AI influences behavior is no longer optional — it’s essential for shaping impactful customer journeys, internal transformation, and strategic positioning.
Who Trusts Algorithms? A Study Overview
The research study conducted by our Chief AI Officer, Vinicius, titled “Trust in the machine: How contextual factors and personality traits shape algorithm aversion and collaboration” and a following article on Psychology Today, co-authored by Peter Slattery explores one of the most nuanced dimensions of AI: trust. Titled “Algorithm Aversion: Who Trusts Algorithms to Make Decisions?”, the piece unpacks why many resist relying on algorithms, even when they outperform humans.
The research-backed article breaks down this resistance — known as algorithm aversion — into understandable factors that any executive can use to shape better digital strategies and communications.
1. Age and Gender Differences in AI Trust
The study found that older individuals are generally more cautious when it comes to algorithmic decision-making. They’re more likely to withdraw trust after even a minor AI error. This insight is crucial when tailoring enterprise solutions or messaging toward mixed demographics in a client base or employee cohort.
Actionable Insight:
To increase adoption among groups more prone to algorithm aversion — such as older individuals — it’s critical to design AI interfaces that offer human override options and explainability features. These users are more likely to disengage when an algorithm makes an error, even a minor one. By embedding features that allow users to understand the “why” behind a decision and optionally choose a manual path, you foster a greater sense of control and trust.
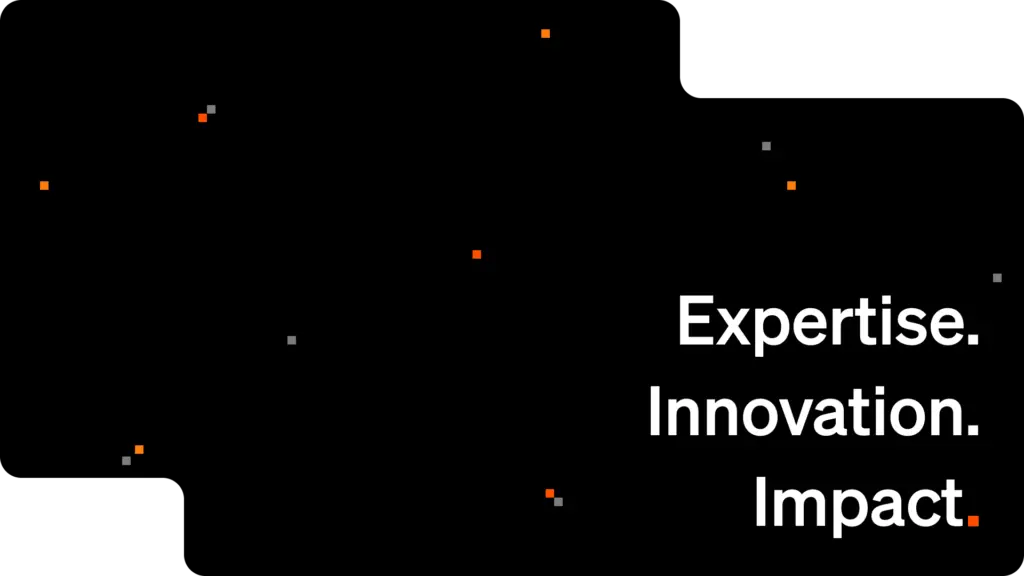
Example 1: Healthcare Industry
Consider a healthcare AI platform recommending treatment options. If an older user sees a suggestion they don’t fully understand, they may be hesitant to accept it. However, if the system includes a “Why this recommendation?” button that breaks down the decision using familiar terms — like previous medical history, success rates, or doctor-approved protocols — and a “Talk to a specialist instead” option, it empowers the user. This approach reassures them that AI supports, rather than replaces, their autonomy, making them more open to future AI-driven interactions.
Discover how we support companies in the Healthcare and Life Science sector ⇲
Example 2: Finance Industry
In the finance industry, think of an AI-powered investment tool that recommends a portfolio shift. An older or more risk-averse investor might resist the recommendation due to a lack of clarity. If the platform includes a “Show my risk profile and why this fits” feature and allows toggling between AI-guided and manual investing options, it enhances both confidence and control. By explaining, for instance, that the recommendation is based on market trends, their past performance preferences, and risk tolerance settings, the tool builds trust while educating the user. A win-win for adoption and loyalty.
2. The Personality Factor: Extraversion & Trust
Personality traits significantly influence how people perceive and interact with AI. The research shows that individuals who are more extraverted, meaning they’re sociable, expressive, and outgoing, tend to be more receptive to AI recommendations. Similarly, people who naturally have a higher baseline of interpersonal trust are less likely to be skeptical of algorithmic decisions.
Why does this matter? Extraverts are often influenced by social proof: they care about what others are doing or saying. Likewise, high-trust individuals don’t feel the same need to question or control every aspect of an AI system’s decision-making process.
Marketing Tip:
To appeal to these user types, incorporate testimonials, case studies, and endorsements from trusted sources or well-known brands. Extraverts and high-trust users respond positively when they see that others have successfully used and benefited from AI. Highlighting peer adoption and positive outcomes can reduce friction and increase engagement.
EXAMPLE
Imagine you’re marketing an AI-driven productivity assistant for executives. You might include a testimonial from a well-known CEO:
Since using this AI assistant, I’ve reduced email time by 40% — and I trust it to keep me on track.
This kind of validation taps into the extraverted and high-trust audience by showing real-world success, which increases their willingness to try it themselves.
3. Transparency as a Key to Adoption
Transparency is a foundational pillar of AI trust. When users don’t understand how an AI arrives at a recommendation or decision, they’re more likely to distrust or reject it — regardless of how accurate it is. This is especially true in high-stakes environments like finance, healthcare, or hiring, where the cost of a “mystery mistake” feels too risky.
Transparent systems demystify the process, providing users with clear, non-technical explanations of what the AI is doing and why. This not only builds trust but also helps users feel confident and in control.
Marketing & UX Tip:
Frame AI offerings with digestible insights into how decisions are made. Use analogies, visuals, or interactive explainers to demystify.
Use “Explainability by design.” Incorporate simple, user-friendly interfaces that include info panels, “Why this?” tooltips, or short narrative summaries explaining how decisions were reached. Think less technical jargon, more practical insight.
EXAMPLE:
A legal tech firm using AI to suggest relevant case law might offer a side panel saying:
This case was selected because it aligns with your search for contract disputes in the financial sector between 2018–2022 and has a 92% citation relevance score.
This approach helps the user connect the dots and trust the AI’s logic, reducing friction and increasing perceived value.
4. The Fear of Error and Perceived Cost
Even when people know AI performs better overall, they often focus disproportionately on what happens when it fails — especially if a mistake could carry significant financial, reputational, or personal costs.
This “single error panic” can lead users to disengage entirely after just one bad experience. Fear-based aversion is common in sectors where risk mitigation is part of the job, like legal, finance, healthcare, and operations.
Marketing & UX Best Practice:
Offer trial periods, sandbox environments, or opt-in AI controls to ease people into trust and build confidence over time.
Mitigate the fear by promoting fail-safes, low-risk trial options, or tiered decision-making models (where AI suggests but doesn’t finalize decisions). Position the AI as assistive, not authoritative — a tool to enhance, not override, human judgment.
EXAMPLE:
A fintech company might offer AI-based credit scoring tools for loan officers. To reduce anxiety, the interface could show:
AI recommendation: Approve. Confidence level: 87% based on income stability, payment history, and current debt ratio.
Below that, include buttons: “Review manually”, “View data inputs”, and “Override with notes.”
This empowers users to trust without surrendering control, keeping them engaged even when the AI isn’t perfect.
5. The Role of Customization and Control
People are far more likely to trust and engage with AI tools that adapt to their preferences, goals, and style of working. Static or one-size-fits-all models can feel foreign, impersonal, or inflexible — especially in leadership or expert roles.
When users can customize how AI interacts with them, it triggers a sense of ownership, personalization, and empowerment. This reduces resistance and helps the AI feel like a collaborative partner rather than an intrusive outsider.
Marketing & UX Tip:
Design for modularity. Let users set thresholds, adjust alert settings, toggle automation levels, or prioritize different outcomes. The goal is to create a guided experience, not a locked one.
EXAMPLE:
In a sales enablement platform using AI to recommend next steps in deal cycles, offer settings like:
- Only notify me if deal risk rises above 60%.
- Prioritize clients with a >$100k pipeline.
- Show me the top 3 actions, not all 10.
This type of control turns AI into a smart assistant that speaks their language, boosting both trust and efficiency.
6. Empirical Evidence Drives Executive Buy-in
Executives trust data. AI adoption skyrockets when solutions are backed by real-world metrics, performance KPIs, and ROI evidence.
Pitch Strategy: Include side-by-side comparisons showing human vs. AI outcomes, productivity improvements, and cost-savings metrics.
7. Strategic Communication: Lessons for Marketers
These findings can be turned into messaging gold:
Behavior Barrier | Communication Strategy |
Fear of error | “Start small. Zero risk demos to prove it works.” |
Lack of understanding | “Clear explainers. No AI PhD required.” |
Gender/Age discomfort | “Inclusive design. AI that listens before acting.” |
Personality mismatch | “AI your way — adaptable to your leadership style.” |
Practical Applications for Enterprises
- Internal Change: Apply this behavioral science in training teams on AI use, rolling out internal automation, or deploying AI-powered dashboards.
- Client Engagement: Use behavior-based segmentation to tailor messaging in B2B or B2C campaigns.
Case Examples of Behavior Change AI in Action
- Banking: AI-guided financial wellness tools influence smarter spending habits.
- Healthcare: Algorithms improve medication adherence via gentle reminders and reward triggers.
- Retail: AI nudges shoppers to re-engage abandoned carts through subtle personalization.
Recommendations for C-Suite Leaders
- Normalize Explainability: Make transparent AI a standard, not an option.
- Tailor for Personality Profiles: Use audience segmentation to design or market AI by behavior type.
- Frame AI as Empowerment: Avoid language that suggests AI “takes over.” Emphasize assistance.
- Offer Low-Risk Onboarding: Use trials, demos, and simulations to build confidence.
- Track Behavior Shifts: Monitor not just outputs, but how users interact with and respond to AI.
❓ Frequently Asked Questions
What is algorithm aversion in simple terms?
It’s the tendency of people to distrust AI, especially after it makes a single mistake, even if it usually performs better than humans.
Who is most likely to avoid trusting AI?
Older individuals and women tend to have higher aversion rates, along with people low in extraversion or general trust.
How can we reduce fear of AI in our organization?
Use transparent design, offer opt-outs, run demos, and train employees to see AI as a support tool rather than a threat.
Why is transparency so important in AI adoption?
Understanding builds trust. People are more likely to accept what they can see and grasp.
How does this impact B2B marketing?
You can tailor AI product messaging by audience segment — using transparency, customization, and data to counter hesitance.
Should AI be presented as a decision-maker?
No. It’s more effective to position AI as an assistant or enhancer, allowing human override and control.
Final Thoughts
Behavior change through AI is both a science and a strategy. With deep insights from research by Psychology Today’s Peter Slattery and our ILI Digital’s CAIO Vinicius, leaders can finally align technology with human psychology to drive real adoption and impact.
For C-level executives and digital innovators, this is more than an opportunity — it’s a playbook for transformation.
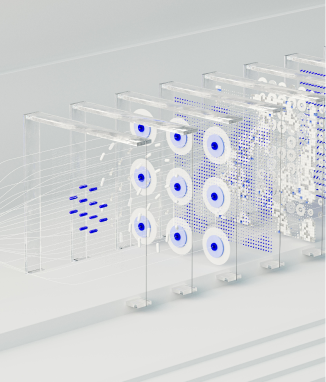